mvpa2.clfs.model_selector.ModelSelector¶
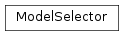
-
class
mvpa2.clfs.model_selector.
ModelSelector
(parametric_model, dataset)¶ Model selection facility.
Select a model among multiple models (i.e., a parametric model, parametrized by a set of hyperparamenters).
Methods
max_log_marginal_likelihood
(hyp_initial_guess)Set up the optimization problem in order to maximize the log_marginal_likelihood. solve
([problem])Solve the maximization problem, check outcome and collect results. TODO:
Methods
max_log_marginal_likelihood
(hyp_initial_guess)Set up the optimization problem in order to maximize the log_marginal_likelihood. solve
([problem])Solve the maximization problem, check outcome and collect results. -
max_log_marginal_likelihood
(hyp_initial_guess, maxiter=1, optimization_algorithm='scipy_cg', ftol=0.001, fixedHypers=None, use_gradient=False, logscale=False)¶ Set up the optimization problem in order to maximize the log_marginal_likelihood.
Parameters: parametric_model : Classifier
the actual parameteric model to be optimized.
hyp_initial_guess : numpy.ndarray
set of hyperparameters’ initial values where to start optimization.
optimization_algorithm : string
actual name of the optimization algorithm. See http://scipy.org/scipy/scikits/wiki/NLP for a comprehensive/updated list of available NLP solvers. (Defaults to ‘ralg’)
ftol : float
threshold for the stopping criterion of the solver, which is mapped in OpenOpt NLP.ftol (Defaults to 1.0e-3)
fixedHypers : numpy.ndarray (boolean array)
boolean vector of the same size of hyp_initial_guess; ‘False’ means that the corresponding hyperparameter must be kept fixed (so not optimized). (Defaults to None, which during means all True)
Notes
The maximization of log_marginal_likelihood is a non-linear optimization problem (NLP). This fact is confirmed by Dmitrey, author of OpenOpt.
-
solve
(problem=None)¶ Solve the maximization problem, check outcome and collect results.
-